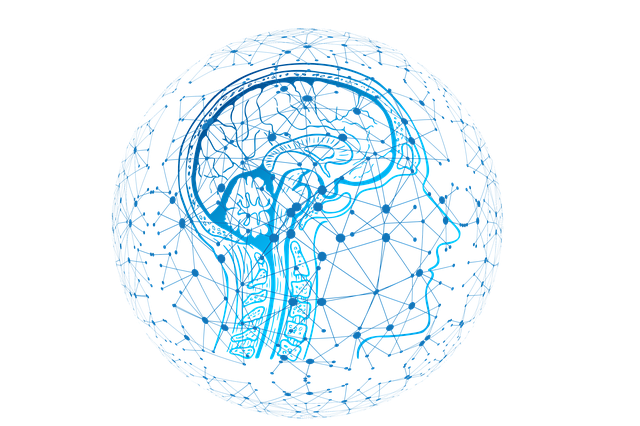
Lots has been written about the risks and challenges in relation to artificial intelligence solutions including the risk of bias. There hasn’t been so much written that specially explores these risk in relation to the use of artificial intelligence solutions within education. As such I would like to share some thoughts on this starting specifically with the risk of bias and how this might impact on education, teachers and students.
Bias in AI systems
AI systems will generally be provided with training data which is then used by the system in generating its output. The quality of this training data therefore has a significant impact on the usefulness of the resulting AI solution. If we provide the system with biased training data, such as an unrepresentative amount of training data relating to a specific event, group or other category, this will result in a biased output. An easy example of this relates to the poor ability for AI based facial recognition systems to identify people of colour. This likely relates to the fact these solutions were created by largely western white individuals who therefore used training data which had an unrepresentative number of western white faces. The challenge however is that humans tend to be biased, albeit often subconsciously, so therefore there it is almost guaranteed that some bias will be intrinsic in the training data provided, but that this bias may be difficult for us to identify.
So what might the impact be in relation to education?
Recommendation Systems
One of the areas where AI has been used for some time is in recommendation systems such as Google Search or the “you might like” on shopping sites like Amazon. We will likely see similar systems in education which will recommend subjects or topics for students to study or may even recommend future study paths from secondary into FE and then onwards into HE. But what if these solutions include bias? I would suspect a gender bias would be the most likely to occur in the first instance, as the AI solution tries to mirror the real world training data it will have been provided, where the real world itself still continues to be biased, advantaging males over females. This would also cause a significant problem in relation to how AI systems might respond to individuals which identify as non-binary given there would be little training data relating to non-binary individuals. What suggestions would it provide when the vast majority of data it has related to males or females only?
Learning Systems
Expanding on recommendation systems, we also will have learning systems which gather data on students as they interact with learning material, providing real time feedback and support, plus guiding students through learning materials specifically selected to meet the needs of the individual student. It will not be obvious how these systems arrive at their output however this output might include selecting content based on its difficulty or challenge level, or providing support and advice based on the identified needs. What if there is bias in the training data which leads the AI to tend towards providing overly difficult or overly easy content to a specific subset of users? Note, this subset of users could be as simple as a gender, users in a specific location or ethnicity, however more likely will be a complex categorisation that we may not fully understand. The key issue here is that some students would be receiving more or less challenging learning content, or more or less support or advice as a result of biased decision making within the artificial intelligence solution. How might this impact on students, their learning and their achievement?
Academic stagnation
Again, building on the above, we need to recognise that AI solutions are probability based. They use the training data provided and then use probability based decision making to identify their outputs and actions. This use of probability means that output and decisions tend towards the average and the statistically most likely. In terms of education this might mean that AI solutions will equally tend to reinforce the average so students in a school where previous students have done historically below national average may be supported by AI solutions to achieve similar results, the historical average for the school, even where the individual student ability or even the ability of a given year group is above this national average. Looked at broadly across all education the world over, AI used in teaching and learning, may tend to focus on a global average, which may disadvantage those who are capable of more than this. It may lead towards more equitable access to education, but it may also lead to a stagnation as all educational efforts tend towards an average.
Divergence
We touched briefly on this earlier, but it also relates to stagnation and a tendency towards the average. AI solutions are provided training data and make decisions based on this, so there is a tendency towards an average but what if students diverge from this average? The lack of data specifically relating to these individuals will mean the AI will tend towards the probable and providing advice or directly students according to how the “average” student might perform, which may be inappropriate for these divergent students. Consider an AI based learning platforming selecting content and providing advice based on the “average” student but where the student using the system is neuro-divergent? Is the content and advice likely to be appropriate for these students? What might the impact on the student, on learning, on their mental health, where being presented with inappropriate learning path ways, support and advice?
Reinforcing Bias
Where AI solutions are generating the learning content themselves based on individual students needs we also need to be conscious of how this might result in the reinforcement of stereotypes and bias. What if the AI solution has to create an image for a criminal, a nurse, or childminder or lawyer; Is there the potential for the images the AI presents to reinforce gender, ethnic or other biases which already exist, and therefore which are highly likely to exist in the training data?
Conclusion
Based on the above it is clearly right to consider the above risks. We need to be conscious of these risks such that we can try to mitigate against them by carefully reviewing the training data being used, and by ongoing review of AI performance. We also need to consider where in some circumstances it may be necessary to have separate AI solutions, with separate training data, for use in certain situation. Although these risks need to be considered we also need to remember that in the absence of AI solutions in education, it has been humans which have made these decisions. And humans aren’t devoid of bias, we just happen to largely be unconscious to it. It is easier to identify bias, or other incorrect or irrational behaviours in others, including in AI systems, than it is to identify it in ourselves. We therefore need to be careful to avoid holding AI up to standards that we ourselves have never been able to meet.
I wonder whether in seeking to address bias in AI solutions the first thing we may need to do is step back and acknowledge the extent of our own human bias both individually and collectively.